DevOps / DevSecOps – Rapid Application Development
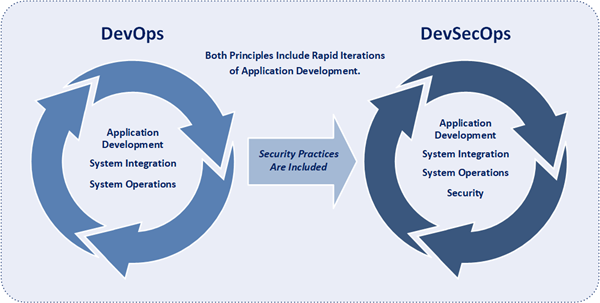
About DevOps
DevOps is a software development paradigm that integrates system operations into the software development process. Moreover, DevOps is the combination of application development, system integration, and system operations. With DevOps development and technical operations personnel collaborate from design through the development process all the way to production support.
Dev is short for development and includes of all the personnel involved in directly developing the software application including programmers, analysts, and testers. Ops is short for operations and includes all personnel directly involved in systems and network operations of any type including systems administrators, database administrators, network engineers, operations and maintenance staff, and release managers.
The primary goal of DevOps to enable enhanced collaboration between development and technical operations personnel. Benefits include more rapid deployment of software applications, enhanced quality of software applications, more effective knowledge transfer, and more effective operational maintenance.
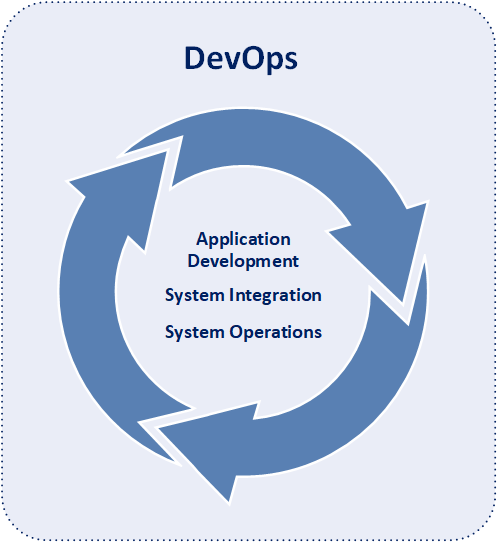
A fundamental practice of DevOps is the delivery of very frequent but small releases of code. These releases are typically more incremental and rapid in nature than the occasional updates performed under traditional release practices. Frequent but small releases reduce risk in overall application deployments. DevOps helps teams address defects very quickly because teams can identify the last release that caused the error. Although the schedule and size of releases will vary, organizations using a DevOps model deploy releases to production environments much more often than organizations using traditional software development practices.
The essential concepts that make DevOps an effective software development approach are collaboration, automated builds, automated tests, automated deployments, & automated monitoring. Moreover, the inclusion of automation into DevOps fosters speed, accuracy, consistency, reliability, and speed of release deployments. Within DevOps, automation is utilized at every phase of the development life cycle starting from triggering of the build, carrying out unit testing, packaging, deploying on to the specified environments, carrying out build verification tests, smoke tests, acceptance test cases and finally deploying on to a production environment. Additionally within DevOps, automation is also included in operations activities, including provisioning servers, configuring servers, configuring networks, configuring firewalls, and monitoring applications within the production environments.
About DevSecOps
DevSecOps is a software development paradigm of integrating security practices into the DevOps process. SecOps is short for security operations and includes the philosophy of completely integrating security into both software development and technical operations as to enable the creation of a “Security as Code” culture throughout the entire IT organization. DevSecOps merges the contrasting goals of rapid speed of delivery and the deployment of highly secure software applications into one streamlined process. Evaluations of the security of code are conducted as software code is being developed. Moreover, security issues are dealt with as they become identified in the early parts of the software development life cycle rather than after a threat or compromise has occurred.
DevSecOps reduces the number of vulnerabilities within deployed software applications and increases the organization’s ability to correct vulnerabilities.
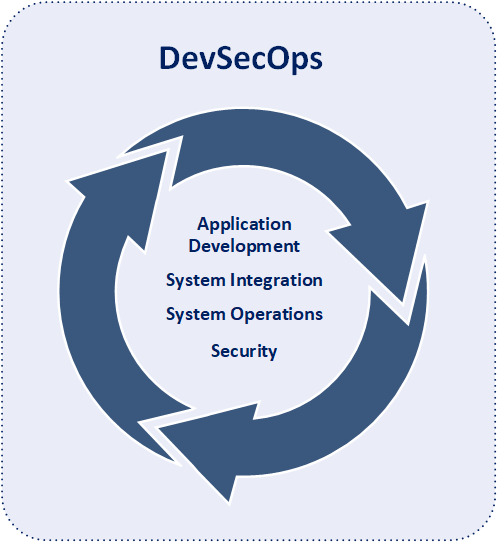
Before the use of DevSecOps, organizations conducted security checks of software applications at the last part of the software development life cycle. By the time performed security checks were performed, the software applications would have already passed through most of the other stages and would have been almost fully developed. So, discovering a security threat at such a late stage meant reworking large amounts of source code, a laborious and time-consuming task. Not surprisingly, patching and hot fixes became the preferred way to resolved security issues in software applications.
DevSecOps demands that security practices be a part of the product development lifecycle and be integrated into each stage of the development life cycle. This more modern development approach enables security issues to be identified and addressed earlier and more cost effectively than is possible with a conventional and more reactive approach. Moreover, DevSecOps engages security at the outset of the development process, empowers developers with effective tools to identify and remediate security findings, and ensures that only secure code is integrated into a product release.