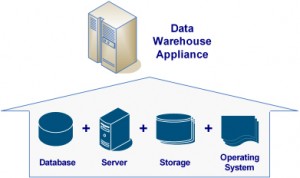
- Complete data warehouse appliances are purpose-built data warehouse solutions and systems that encompass a whole-technology stack including:
- • Operating System (OS)
- • Database Management System (DBMS)
- • Server Hardware
- • Storage Capabilities
Initially DW appliances were created with proprietary custom-built hardware and storage units. Netezza, Teradata, DATAllegro, & White Cross (now Kognito) were the first vendors to provide solutions in this manner. Subsequently data warehouse appliances evolved and started to utilize lower-cost, industry-standard non-proprietary hardware components.The movement from proprietary to commodity hardware has proven to bring down the cost of the data warehouse appliance as the commodity hardware can integrated at a lower cost of both developing and integrating proprietary hardware. Examples of commodity hardware typically include general-purpose servers from Dell, Hewlett Packard (HP), or IBM utilizing Intel processors and popular network and storage hardware from either Cisco, EMC, or Sun.
Introduced 2002, Netezza was the first vendor to offer a complete data warehouse appliance, so early definitions of appliance were based upon Netezza products. Subsequently, Netezza Performance Server still provides all of the software components of a data warehouse appliance, including the database, operating system, servers, and storage units. However in 2009, Netezza replaced its own proprietary hardware with IBM blade servers and storage units. Further in 2010, IBM completed a corporate acquisition of Netezza.
Similar to Netezza, DATAllegro was launched in 2005 with a complete solution involving proprietary hardware. Soon after DATAllegro replaced its own proprietary hardware with commodity server from Dell and storage units from EMC. In 3008, Microsoft acquired DATAllegro in 2008 and announced it will integrate DATAllegro’s massive parallel processing (MPP) architecture into its own MS SQL Server platform, which also runs on commonly-available hardware.
Additionally, both Kognitio and Teradata replaced their proprietary hardware within their appliances in a process similar to that of DATAllegro. Kognitio now offers a row-based, in-memory database database called WX2 that does not include indexes or data partitions and runs on blade servers from IBM and Hewlett-Packard. Teradata provides a proprietary database, a variety of common operating systems (Linux, Unix, and Windows), and a proprietary networking subsystem packaged along with commodity processors and storage units.
Announced at the 2008 Oracle OpenWorld conference in San Francisco, the Oracle Exadata Database Machine is a complete package of database software, operating system, servers, and storage. The product was initially assembled in collaboration between Oracle Corporation and Hewlett Packard where Oracle developed the database, operating system and storage software, while HP constructed the hardware. However, with Oracle’s acquisition of Sun Microsystems, Oracle announced the release of Exadata Version two with improved performance and usage of Sun Microsystems storage and operating systems technologies.
At the Sapphire conference in May, 2010 in Orlando, SAP announced the release of its new data warehouse appliance called HANA or High-Performance Analytic Appliance. SAP HANA is a combination of hardware, storage, operating system, management software, and in-memory data query engine that is characterized by data being held in RAM rather than being read from disks or flash storage.
Finally IBM bundles and integrates its own Infosphere Warehouse database software (formerly “DB2 Warehouse”) with its own servers and storage to deliver the IBM Infosphere Balanced Warehouse.