Type Two – Update Record to Inactive / Create an Active Record
Type two modeling is a very reliable and straightforward technique for preserving history of changes to dimensional tables. It is commonly utilized when a full or partial set of the previous values of a dimension’s attributes must be retained. In type two modeling, every time an update occurs to any of the values in a dimensional table, a new record is physically inserted as active or current into the same table and the old records are marked inactive or historical.
The main advantage of this modeling technique is that it can accommodate and record a massive amount of history and nearly an unlimited changes to slowly changing dimensions. But this advantage also can become a significant drawback. Within this modeling technique, it is possible for dimension tables to grow to massive sizes and adversely affect both system and query performance. In addition, since upon every change this modeling technique requires an update to the linked fact table, implementation is fairly difficult and may require substantial effort to design, develop, and support.
Two unique sub-methods have been established for distinguishing the active (or current) record from the inactive (or historical) records: active flagging and tuple versioning.
In the type two – active flagging sub-method, an “Active” column is constructed within the dimensional table and acts as a flag. Flags are binary fields with permissible values being Y and N, T and F, or 1 and 0. A positive value (Y, T, 1) indicates an active record, while a negative value (N, F, 0) indicates an inactive record.
Example of Type Two – Active Flagging Sub-Method
Suppose that a vendor changes his phone number to 858-555-6555 from 202-555-8639 because the phone company has added a new area code. Using active flagging, the change to the vendor dimension table would be processed as follows…
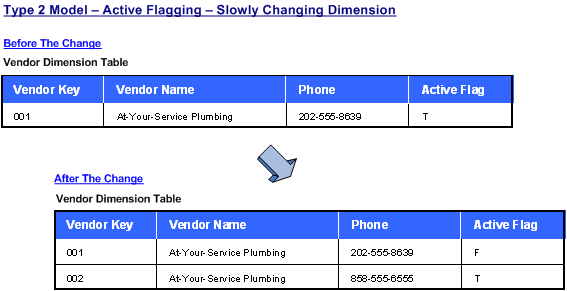
- • The current active record in the vendor dimension table is updated:
- – The active flag is changed from T to F.
- – The phone number field remains as 202-555-6555.
- • A new record is inserted into the vendor dimension table:
- – The vendor key is given the next available integer number.
- – The vendor name field remains the same as in the old record.
- – The phone number has the new value of 858-555-8639.
- – The active flag is set to T.
In the type two – tuple versioning sub-method, start date and end date columns are included on the dimension table. The values of these date fields then define the period during which that record has been active. For the most part, the start date is the date the record has been created. Moreover, the end date is the date the record has become inactive, either because a newer record has replaced it or because the original record in the source system no longer exists. On the active record, the end date will either be left null, blank, or identified in another way such as all-nines, according to modeler’s preferences and standards.
The main advantage of the tuple versioning method over active flagging is that it provides an audit trail of the date and sequence of all updates.
Example of Type Two – Tuple Versioning Sub-Method
Continuing with the same example above but using the tuple versioning method, the updated phone number in a record in the vendor dimension table is processed as follows:
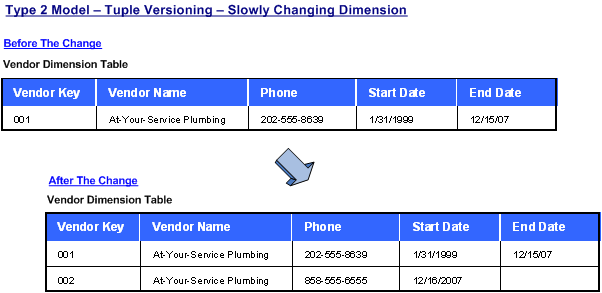
- • The current active record in the vendor dimension table is updated:
- – The end date is updated to the date of the change.
- – The phone number field remains as 202-555-6555.
- • A new record is inserted into the vendor dimension table:
- – The vendor key is given the next available integer number.
- – The vendor name field remains the same as in the old record.
- – The phone number has the new value of 858-555-8639.
- – The start date is the date the record is inserted into the table.
- – The end date is intentionally left blank.